The Impact of Sample Size on Exchangeability in the Bayesian Synthesis Approach to Data Fusion
DOI:
https://doi.org/10.35566/jbds/v2n1/p5Keywords:
Bayesian synthesis, Data fusion, ExchangeabilityAbstract
Data fusion approaches have been adopted to facilitate more complex analyses and produce more accurate results. Bayesian Synthesis is a relatively new approach to data fusion where results from the analysis of one dataset are used as prior information for the analysis of the next dataset. Datasets of interest are sequentially analyzed until a final posterior distribution is created, incorporating information from all candidate datasets, rather than simply combining the datasets into one large dataset and analyzing them simultaneously. One concern with this approach lies in the sequence of datasets being fused. This study examines whether the order of datasets matters when the datasets being fused each have substantially different sample sizes. The performance of Bayesian Synthesis with varied sample sizes is evaluated by examining results from simulated data with known population values under a variety of conditions. Results suggest that the order in which the dataset are fused can have a significant impact on the obtained estimates.
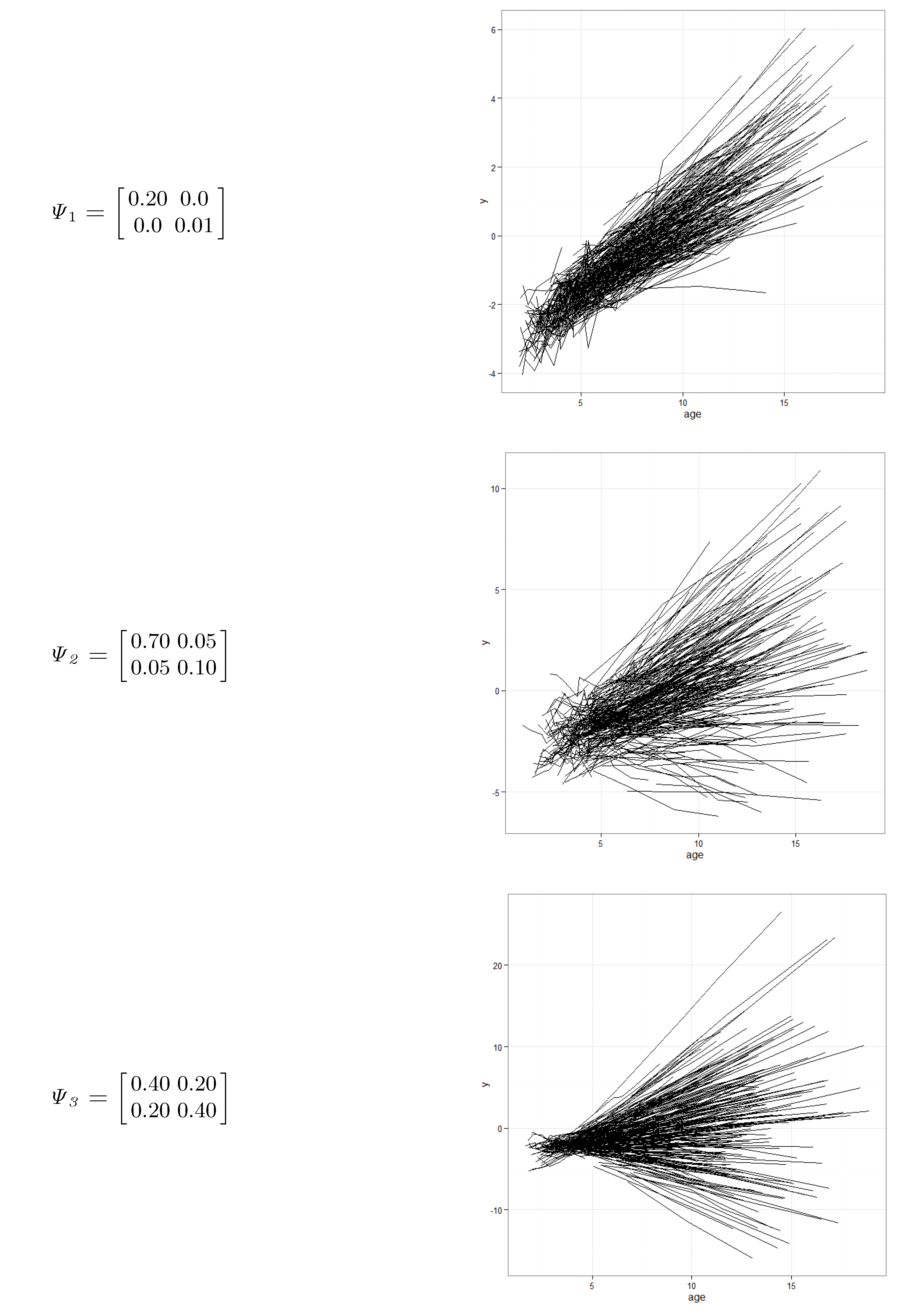
Published
Issue
Section
How to Cite
Accepted %2022-%07-%13
Published %2022-%07-%26