Disentangling the Influence of Data Contamination in Growth Curve Modeling: A Median Based Bayesian Approach
DOI:
https://doi.org/10.35566/jbds/v2n2/p1Keywords:
Robust methods, growth curve modeling, conditional medians, Laplace distributionAbstract
Growth curve models (GCMs), with their ability to directly investigate within-subject change over time and between-subject differences in change for longitudinal data, are widely used in social and behavioral sciences. While GCMs are typically studied with the normal distribution assumption, empirical data often violate the normality assumption in applications. Failure to account for the deviation from normality in data distribution may lead to unreliable model estimation and misleading statistical inferences. A robust GCM based on conditional medians was recently proposed and outperformed traditional growth curve modeling when outliers are present resulting in nonnormality. However, this robust approach was shown to perform less satisfactorily when leverage observations existed. In this work, we propose a robust double medians growth curve modeling approach (DOME GCM) to thoroughly disentangle the influence of data contamination on model estimation and inferences, where two conditional medians are employed for the distributions of the within-subject measurement errors and of random effects, respectively. Model estimation and inferences are conducted in the Bayesian framework, and Laplace distributions are used to convert the optimization problem of median estimation into a problem of obtaining the maximum likelihood estimator for a transformed model. A Monte Carlo simulation study has been conducted to evaluate the numerical performance of the proposed approach, and showed that the proposed approach yields more accurate and efficient parameter estimates when data contain outliers or leverage observations. The application of the developed robust approach is illustrated using a real dataset from the Virginia Cognitive Aging Project to study the change of memory ability.
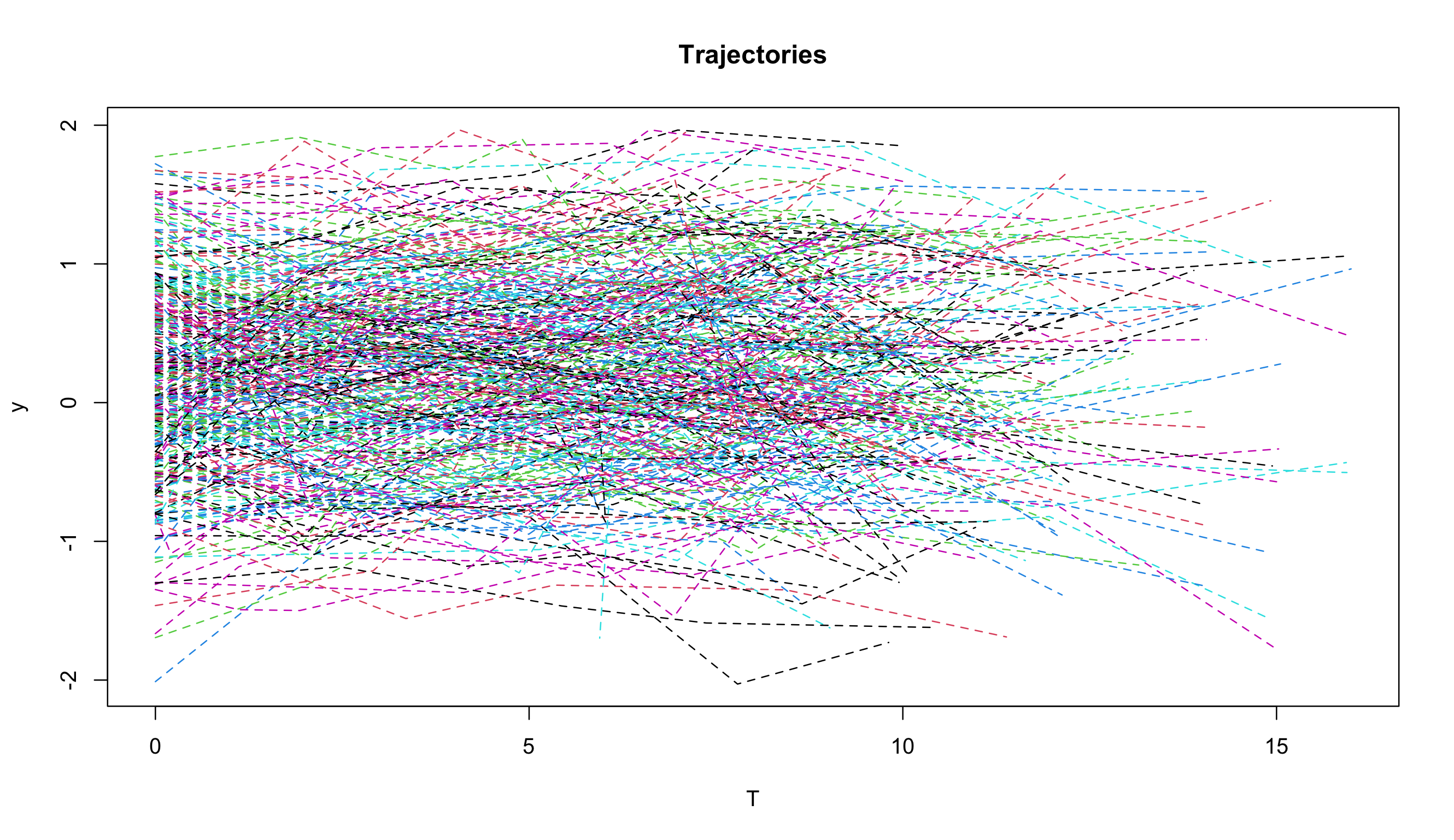