Factor or Network Model? Predictions From Neural Networks
DOI:
https://doi.org/10.35566/jbds/v1n1/p5Keywords:
Neural Networks, Machine Learning, Data Generating MechanismsAbstract
The nature of associations between variables is important for constructing theory about psychological phenomena. In the last decade, this topic has received renewed interest with the introduction of psychometric network models. In psychology, network models are often contrasted with latent variable (e.g., factor) models. Recent research has shown that differences between the two tend to be more substantive than statistical. One recently developed algorithm called the Loadings Comparison Test (LCT) was developed to predict whether data were generated from a factor or small-world network model. A significant limitation of the current LCT implementation is that it's based on heuristics that were derived from descriptive statistics. In the present study, we used artificial neural networks to replace these heuristics and develop a more robust and generalizable algorithm. We performed a Monte Carlo simulation study that compared neural networks to the original LCT algorithm as well as logistic regression models that were trained on the same data. We found that the neural networks performed as well as or better than both methods for predicting whether data were generated from a factor, small-world network, or random network model. Although the neural networks were trained on small-world networks, we show that they can reliably predict the data-generating model of random networks, demonstrating generalizability beyond the trained data. We echo the call for more formal theories about the relations between variables and discuss the role of the LCT in this process.
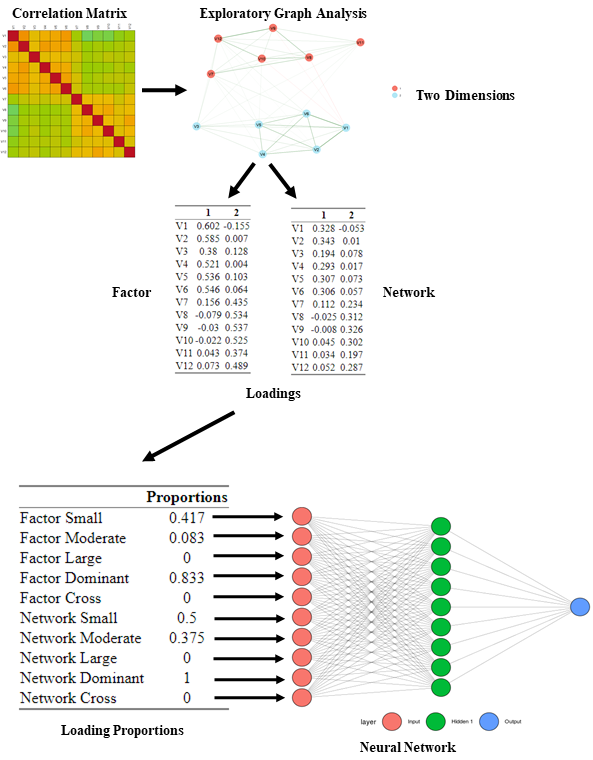