Relative Predictive Performance of Treatments of Ordinal Outcome Variables across Machine Learning Algorithms and Class Distributions
DOI:
https://doi.org/10.35566/jbds/v2n2/suzukiKeywords:
Ordinal classification, Machine learning, Predictive performance, Class imbalance, Measurement scaleAbstract
Abstract Ordinal variables, such as those measured on a five-point Likert scale, are ubiquitous in the behavioral sciences. However, machine learning methods for modeling ordinal outcome variables (i.e., ordinal classification) are not as well-developed or widely utilized, compared to classification and regression methods for modeling nominal and continuous outcomes, respectively. Consequently, ordinal outcomes are often treated “naively” as nominal or continuous outcomes in practice. This study builds upon previous literature that has examined the predictive performance of such naïve approaches of treating ordinal outcome variables compared to ordinal classification methods in machine learning. We conducted a Monte Carlo simulation study to systematically assess the relative predictive performance of an ordinal classification approach proposed by Frank and Hall (2001) against naïve approaches according to two key factors that have received limited attention in previous literature: (1) the machine learning algorithm being used to implement the approaches and (2) the class distribution of the ordinal outcome variable. The consideration of these important, practical factors expands our knowledge on the consequences of naïve treatments of ordinal outcomes, which are shown in this study to vary substantially according to these factors. Given the ubiquity of ordinal measures coupled with the growing presence of machine learning applications in the behavioral sciences, these are important considerations for building high-performing predictive models in the field.
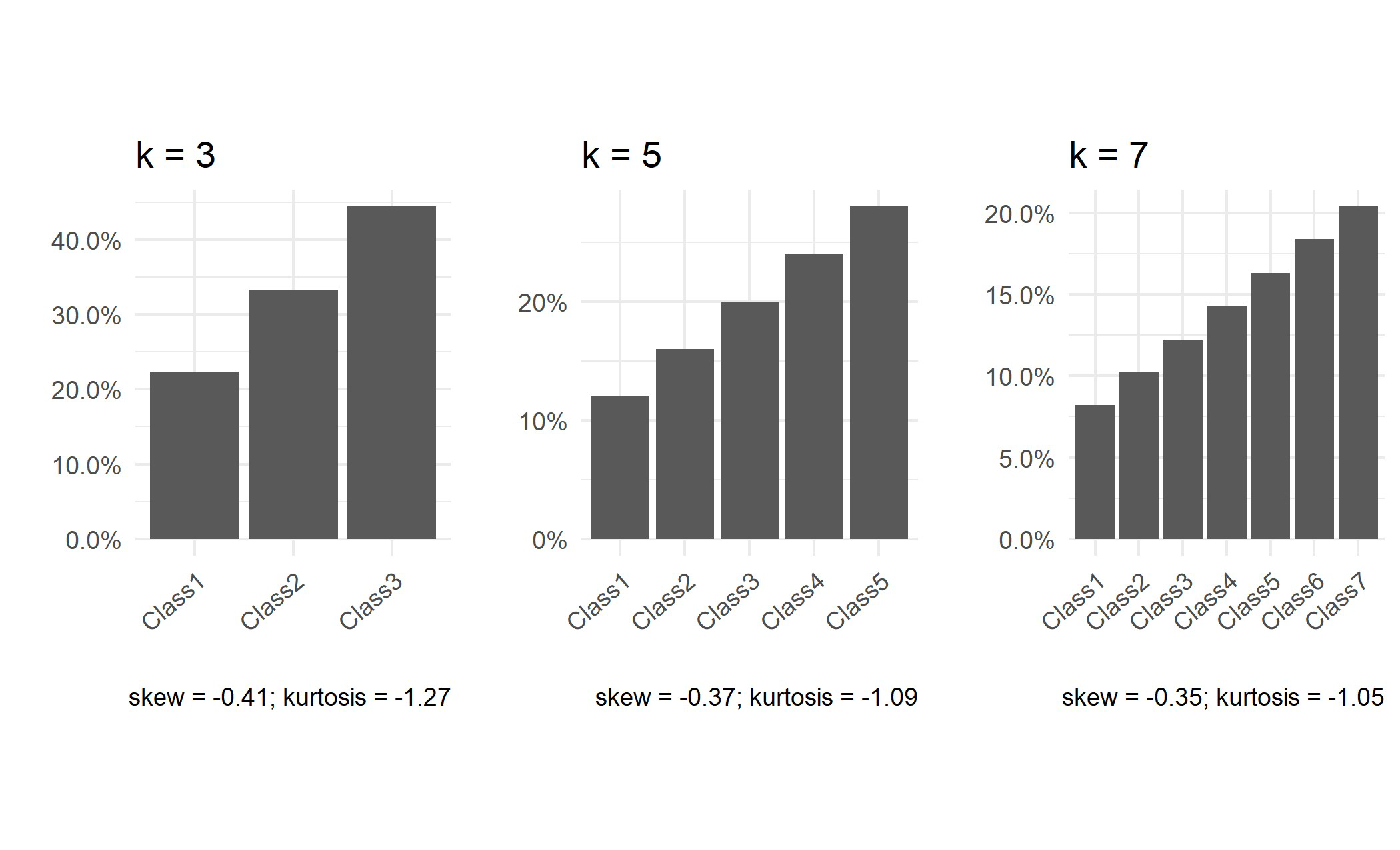
Additional Files
Published
Issue
Section
How to Cite
Accepted %2022-%12-%06
Published %2022-%12-%16