The Performances of Gelman-Rubin and Geweke's Convergence Diagnostics of Monte Carlo Markov Chains in Bayesian Analysis
DOI:
https://doi.org/10.35566/jbds/v2n2/p3Keywords:
Convergence diagnostics , Bayesian analysis, Gelman-Rubin diagnostic, Geweke diagnosticAbstract
Bayesian statistics have been widely used given the development of Markov chain Monte Carlo sampling techniques and the growth of computational power. A major challenge of Bayesian methods that has not yet been fully addressed is how we can appropriately evaluate the convergence of the random samples to the target posterior distributions. In this paper, we focus on Gelman and Rubin's diagnostic (PSRF), Brooks and Gleman's diagnostic (MPSRF), and Geweke's diagnostics, and compare the Type I error rate and Type II error rate of seven convergence criteria: MPSRF>1.1, any upper bound of PSRF is larger than 1.1, more than 5% of the upper bounds of PSRFs are larger than 1.1, any PSRF is larger than 1.1, more than 5% of PSRFs are larger than 1.1, any Geweke test statistic is larger than 1.96 or smaller than -1.96, and more than 5% of Geweke test statistics are larger than 1.96 or smaller than -1.96. Based on the simulation results, we recommend the upper bound of PSRF if we only can choose one diagnostic. When the number of estimated parameters is large, between the diagnostic per parameter (i.e., PSRF) or the multivariate diagnostic (i.e., MPSRF), we recommend the upper bound of PSRF over MPSRF. Additionally, we do not suggest claiming convergence at the analysis level while allowing a small proportion of the parameters to have significant convergence diagnosis results.
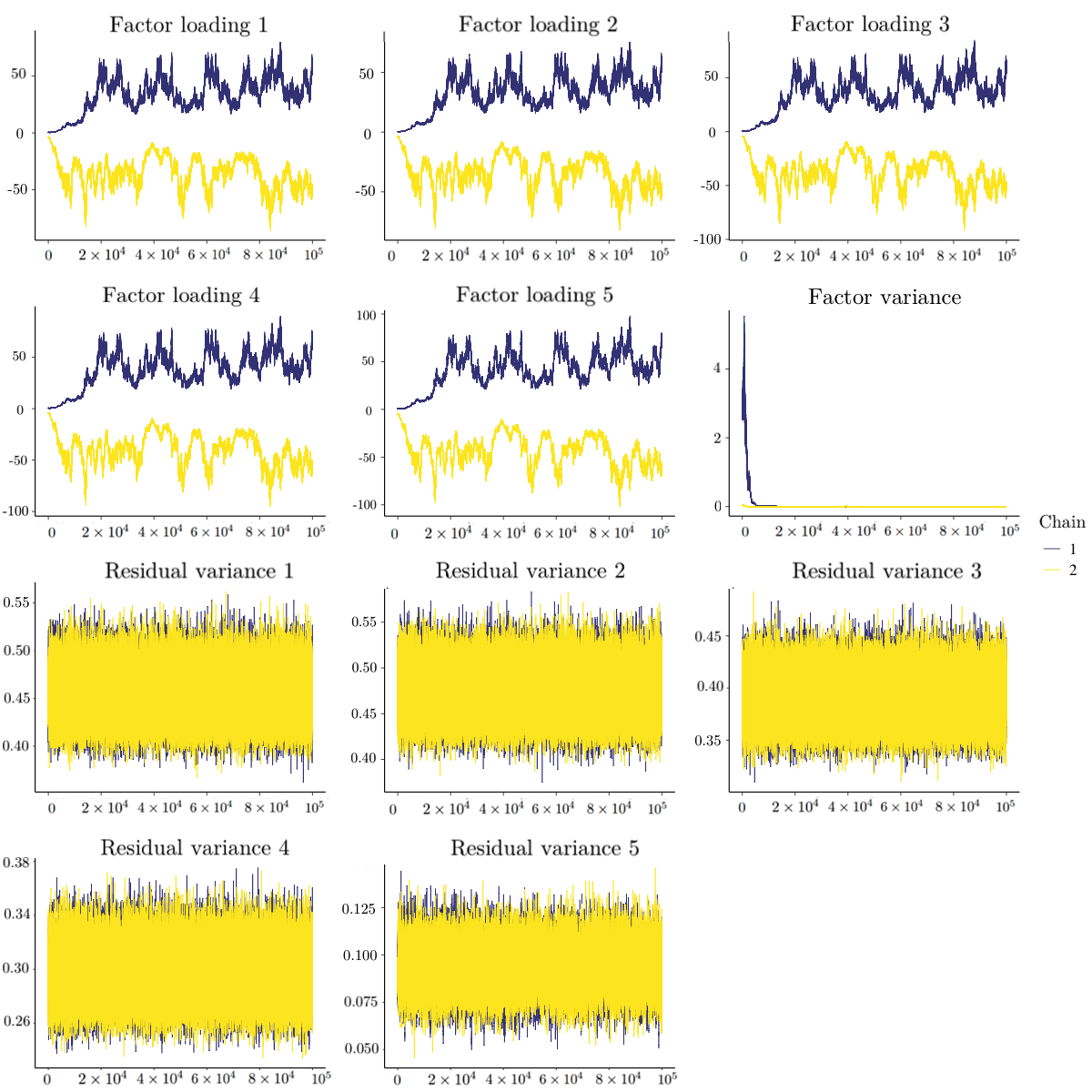