Handling Ignorable and Non-ignorable Missing Data through Bayesian Methods in JAGS
DOI:
https://doi.org/10.35566/jbds/v2n2/xuKeywords:
Missing Data, Bayesian Analysis, Structural Equation ModelingAbstract
With the prevalence of missing data in social science research, it is necessary to use methods for handling missing data. One framework in which data with missing values can still be used for parameter estimation is the Bayesian framework. In this tutorial, different missing data mechanisms including Missing Completely at Random, Missing at Random, and Missing Not at Random are introduced. Methods for estimating models with missing values under the Bayesian framework for both ignorable and non-ignorable missingness are also discussed. A structural equation model on data from the Advanced Cognitive Training for Independent and Vital Elderly study is used as an illustration on how to fit missing data models in JAGS.
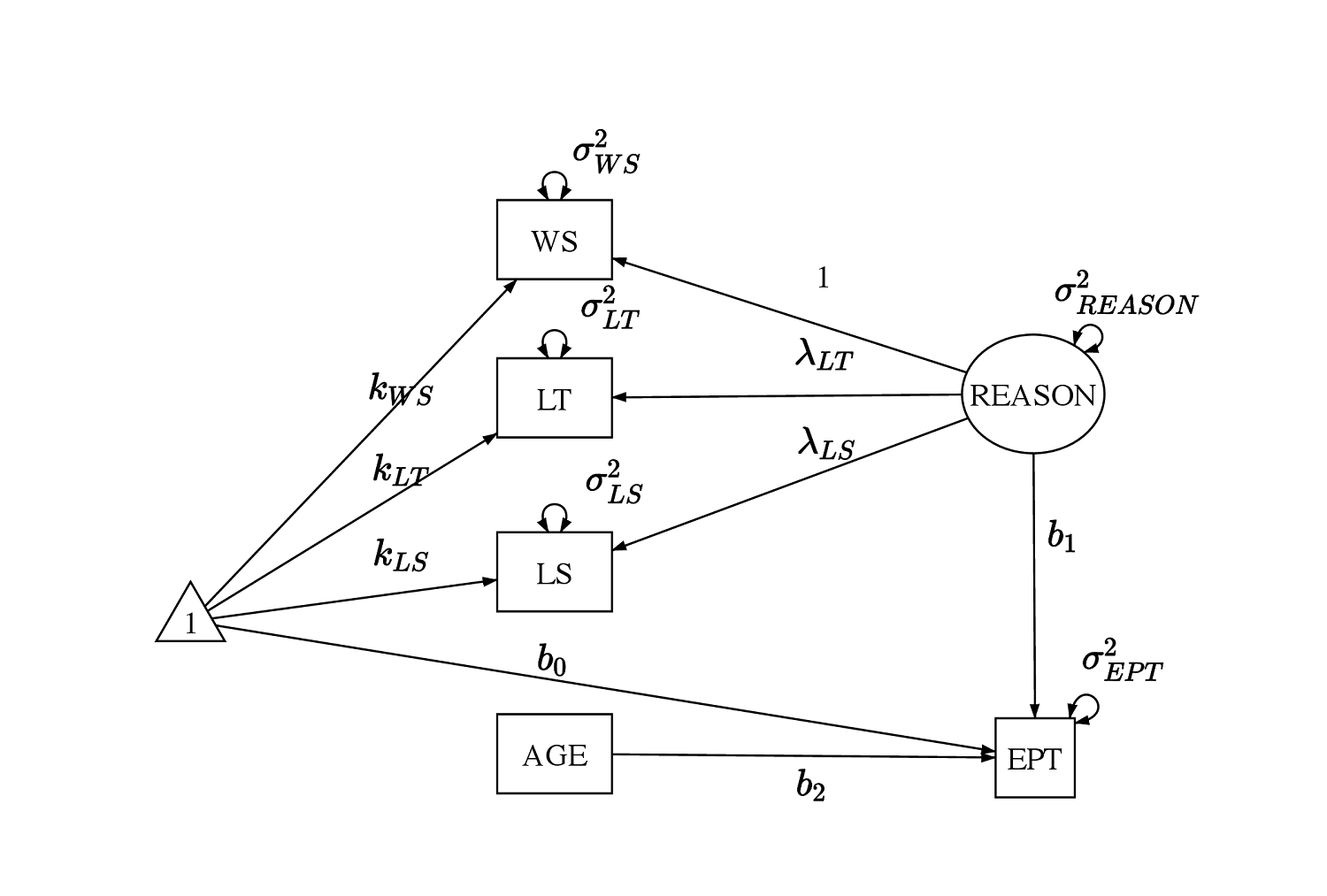