Lasso and Group Lasso with Categorical Predictors: Impact of Coding Strategy on Variable Selection and Prediction
DOI:
https://doi.org/10.35566/jbds/v3n2/montoyaKeywords:
Lasso regression, Categorical predictors, RegularizationAbstract
Machine learning methods are being increasingly adopted in behavioral research. Lasso regression performs variable selection and regularization, and is particularly appealing to behavioral researchers because of its connection to linear regression. Researchers may expect properties of linear regression to translate to lasso, but we demonstrate that this assumption is problematic for models with categorical predictors. Specifically, we demonstrate that while the coding strategy used for categorical predictors does not impact the performance of linear regression, it does impact lasso’s performance. Group lasso is an alternative to lasso for models with categorical predictors. We investigate the discrepancy between lasso and group lasso models using a real data set: lasso performs different variable selection and has different prediction accuracy depending on the coding strategy, while group lasso performs consistent variable selection but has different prediction accuracy. Using a Monte Carlo simulation, we demonstrate a specific case where group lasso tends to include many variables when few are needed, leading to overfitting. We conclude with recommended solutions to this issue and future directions of exploration to improve the implementation of machine learning approaches in behavioral science. This project shows that when using lasso and group lasso with categorical predictors, the choice of coding strategy should not be ignored.
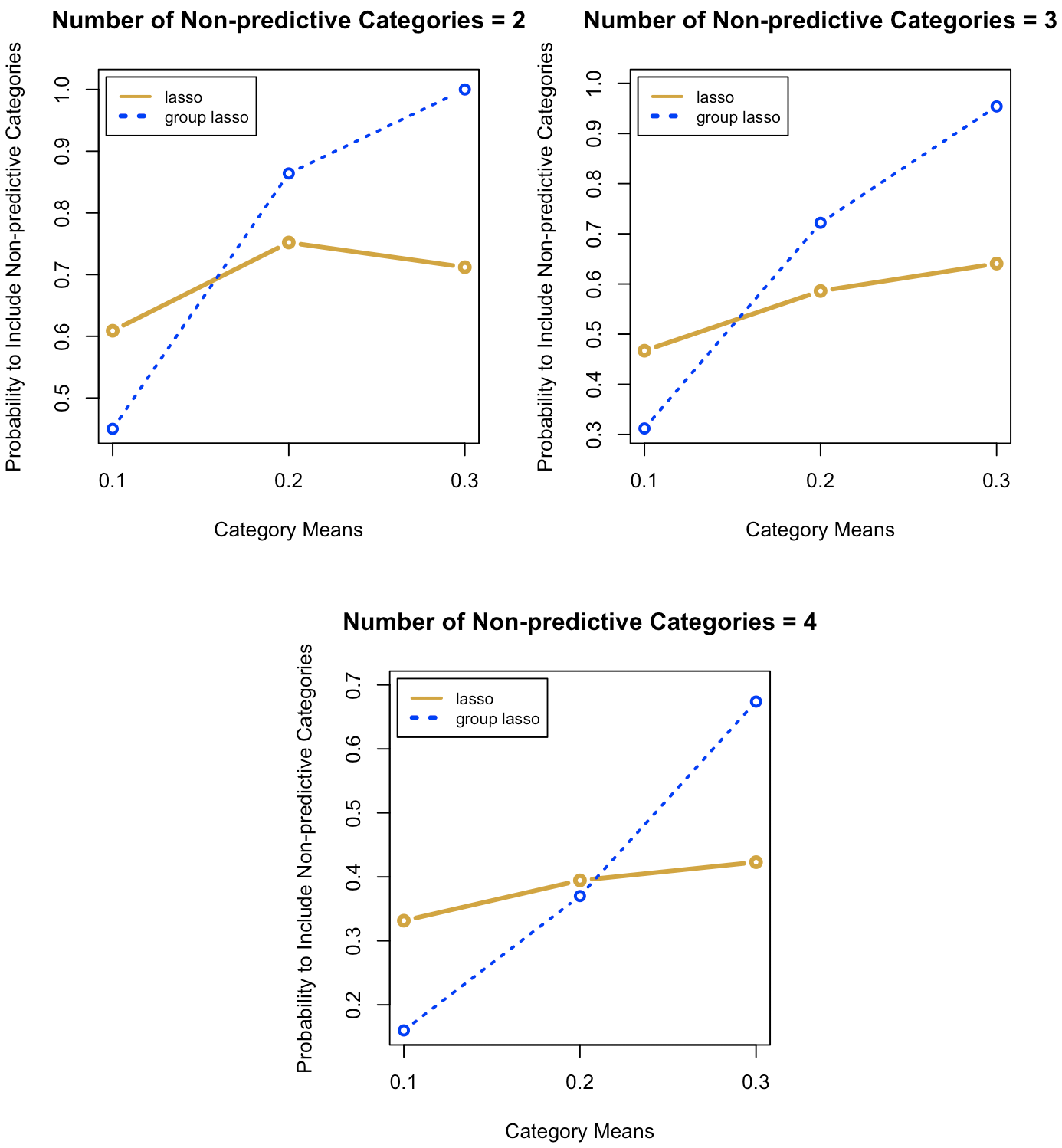