A Novel Approach for Identifying Unobserved Heterogeneity in Longitudinal Growth Trajectories Using Natural Cubic Smoothing Splines
DOI:
https://doi.org/10.35566/jbds/marcoulidesKeywords:
Unobserved heterogeneity, Latent class detection, Natural cubic smoothing splinesAbstract
A novel algorithmic modeling method is proposed to determine dissimilarities between subjects for longitudinal data clustering using natural cubic smoothing splines. Although various modeling techniques have to date been suggested for conducting such analyses, a major problem with many of these approaches is that they often impose overly restrictive assumptions. As a consequence, potentially problematic interpretations of data clustering regarding both the number and the nature of the growth trajectory patterns can occur. The proposed method is shown to be highly effective in identifying heterogeneity of growth trajectories in settings with data exhibiting complex nonlinear longitudinal patterns and without imposing potentially problematic constraints on the model.
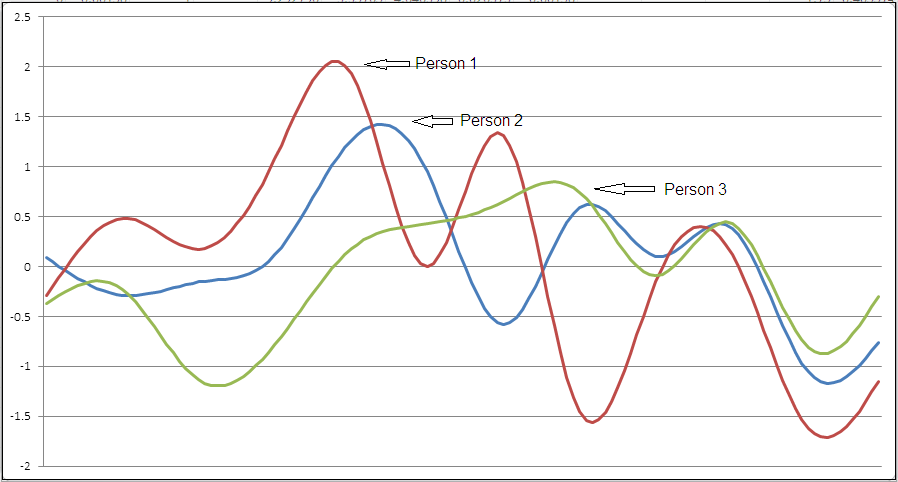