Tree-based Matching on Structural Equation Model Parameters
DOI:
https://doi.org/10.35566/jbds/v1n2/p3Keywords:
matching, structural equation modeling, decision trees, machine learningAbstract
Understanding causal effects of a treatment is often of interest in the social sciences. When treatments cannot be randomly assigned, researchers must ensure that treated and untreated participants are balanced on covariates before estimating treatment effects. Conventional practices are useful in matching such that treated and untreated participants have similar average values on their covariates. However, situations arise in which a researcher may instead want to match on model parameters. We propose an algorithm, Causal Mplus Trees, which uses decision trees to match on structural equation model parameters and estimates conditional average treatment effects in each node. We provide a proof of concept using two small simulation studies and demonstrate its application using COVID-19 data.
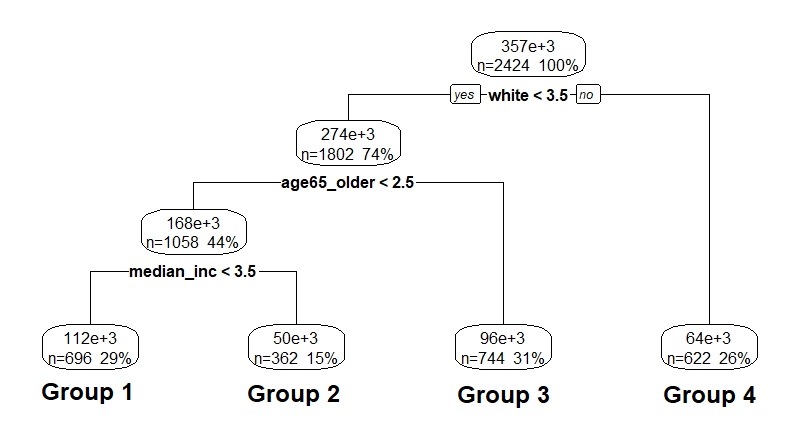