How to Select the Best Fit Model among Bayesian Latent Growth Models for Complex Data
DOI:
https://doi.org/10.35566/jbds/v2n1/p2Keywords:
Model Selection Criterion, Bayesian Estimation, Latent Growth Models, Missing Data, Robust MethodAbstract
Bayesian approach is becoming increasingly important as it provides many advantages in dealing with complex data. However, there is no well-defined model selection criterion or index in a Bayesian context. To address the challenges, new indices are needed. The goal of this study is to propose new model selection indices and to investigate their performances in the framework of latent growth mixture models with missing data and outliers in a Bayesian context. We consider latent growth models because they are very flexible in modeling complex data and becoming increasingly popular in statistical, psychological, behavioral, and educational areas. Specifically, this study conducted five simulation studies to cover different cases, including latent growth curve models with missing data, latent growth curve models with missing data and outliers, growth mixture models with missing data and outliers, extended growth mixture models with missing data and outliers, and latent growth models with different classes. Simulation results show that almost all proposed indices can effectively identify the true model. This study also illustrated the application of these model selection indices in real data analysis.
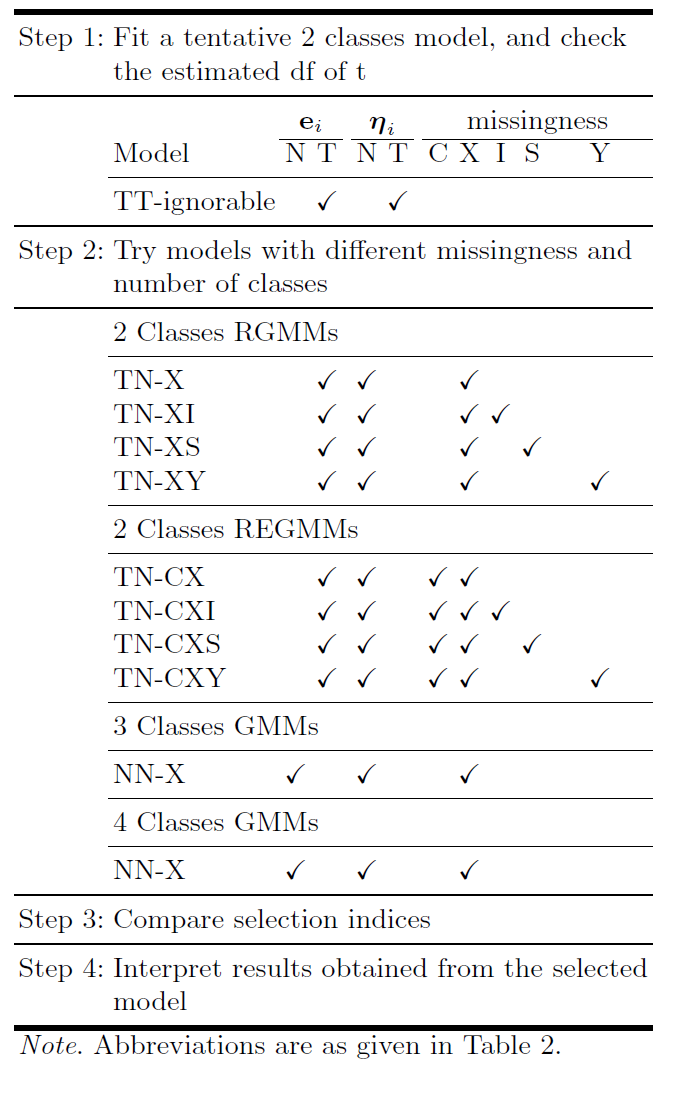